The Essential Guide to Image Labeling Tools for Classification
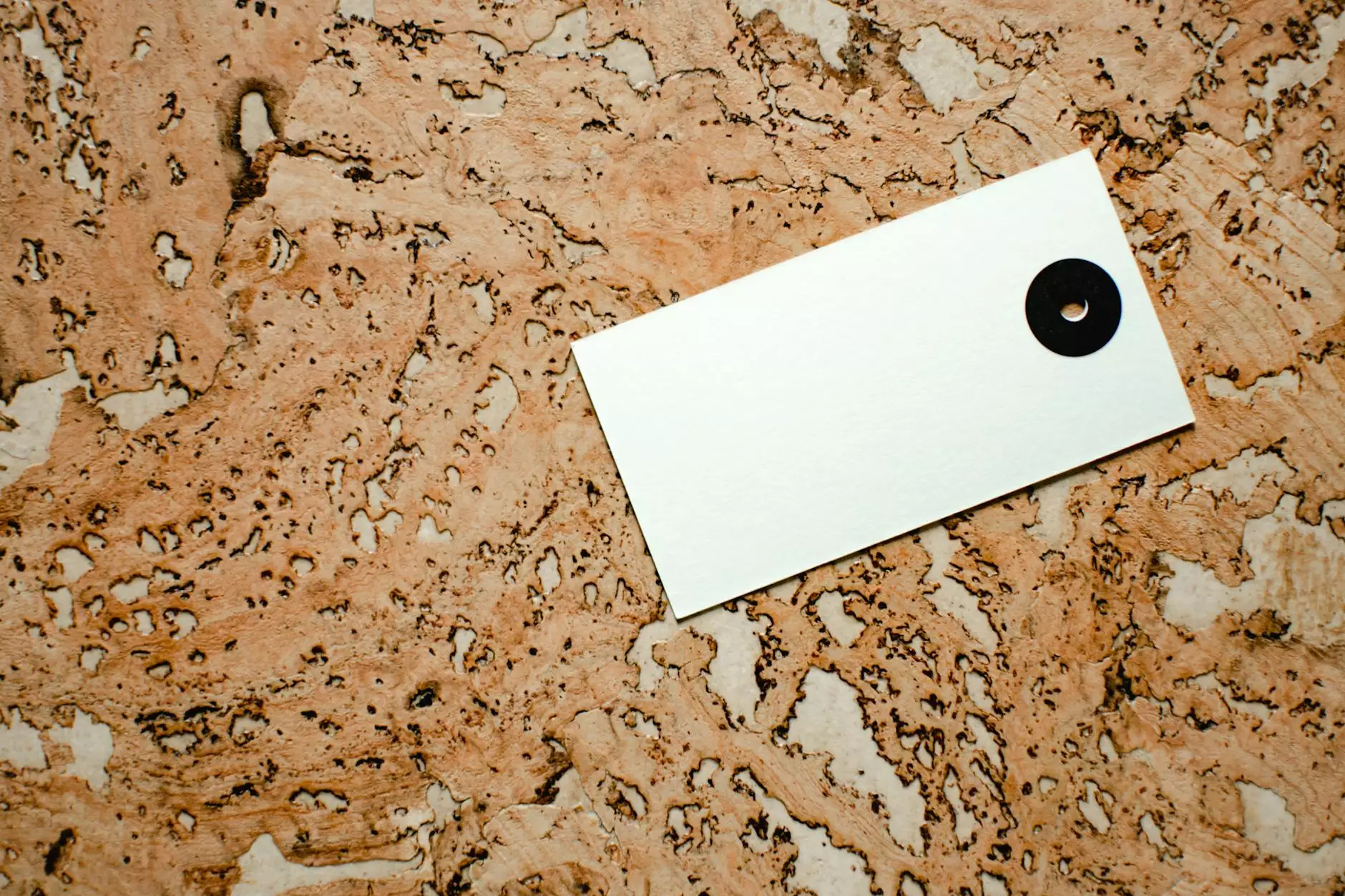
In today's data-driven world, businesses in various sectors are increasingly relying on effective and efficient methods to manage and analyze their data. One such method is through the use of image labeling tools for classification, which play a vital role in organizing and categorizing images for various applications, including machine learning, artificial intelligence, and digital marketing.
What are Image Labeling Tools?
Image labeling tools are specialized software applications designed to annotate images by assigning labels or categories to specific elements within those images. This process is crucial for enabling machines to interpret and understand visual content accurately.
Key Features of Image Labeling Tools
- User-Friendly Interface: Modern tools are designed with intuitive interfaces, making them accessible for users with various skill levels.
- Versatile Annotation Options: These tools often support various annotation types, including bounding boxes, polygons, lines, and semantic segmentation.
- Collaboration Capabilities: Many image labeling tools allow multiple users to collaborate on projects, facilitating teamwork and efficiency.
- Integration with Machine Learning Frameworks: These tools can integrate seamlessly with popular machine learning frameworks, enhancing workflow and productivity.
- Export Options: Once the labeling is complete, users can often export the annotated data in multiple formats for use in various applications.
Importance of Image Labeling for Classification
In fields like machine learning and computer vision, the accuracy of model predictions heavily relies on the quality of the training data. Here are several reasons why image labeling is essential:
1. Data Accuracy
When images are accurately labeled, the classification models trained on this data can achieve higher accuracy. Mislabeling or lack of labeling can lead to poor model performance, directly affecting business decisions and outcomes.
2. Enhanced Machine Learning Outcomes
Image labeling tools significantly enhance the performance of machine learning algorithms by providing high-quality, well-annotated datasets. This leads to better model generalization and robustness in real-world applications.
3. Streamlined Business Operations
By efficiently classifying visual content, businesses can streamline their operations, improve workflow, and enhance overall productivity. This is particularly important for companies dealing with large volumes of images daily.
4. Improved Customer Experience
Businesses that utilize image classification effectively can provide better services to customers by delivering more accurate search results and personalized content, enhancing user engagement and satisfaction.
Popular Image Labeling Tools for Classification
There are various image labeling tools available in the market, each with unique features and capabilities. Below is a list of some of the most popular ones:
- Labelbox: A powerful tool providing an intuitive platform for image annotation with robust collaboration features.
- VGG Image Annotator (VIA): An open-source tool that allows users to label images and export annotations in various formats.
- Roboflow: A comprehensive tool that supports image labeling, dataset management, and model training, making it ideal for end-to-end pipelines.
- RectLabel: A macOS application that allows users to label images for object detection and segmentation, supporting various export formats.
- Supervisely: An online platform that provides advanced image labeling capabilities with extensive support for deep learning frameworks.
How to Choose the Right Image Labeling Tool
When selecting an image labeling tool for classification, it is important to consider several factors to ensure you choose the best fit for your business needs:
1. Purpose and Use Case
Identify the specific requirements of your project. Are you focusing on object detection, segmentation, or classification? Different tools cater to different use cases, so it's crucial to align your choice with your goals.
2. Ease of Use
The tool should have a user-friendly interface that enables both technical and non-technical users to navigate effortlessly. Consider tools that offer tutorials, guides, or customer support to assist with onboarding.
3. Collaboration Features
If your team works collaboratively, look for tools that offer features allowing multiple users to work on the same project simultaneously. This can enhance productivity and efficiency.
4. Integration Capabilities
Choose a tool that integrates with your existing workflows and other tools you are using, such as machine learning frameworks and data storage solutions. This will streamline your processes and reduce friction in operations.
5. Export Options
Check the export options available in the tool. The ability to export labeled data in various formats is essential for compatibility with your machine learning models and data analysis tools.
Implementing Image Labeling in Your Business
Once you have selected the right image labeling tool for classification, the next step is to effectively implement it within your business processes. Here are the steps to guide you:
Step 1: Define Your Goals
Clearly outline what you aim to achieve with image labeling. Whether it’s improving product recommendations or enhancing image searches, having specific goals will guide your labeling strategy.
Step 2: Determine Your Data Sources
Identify the images that need labeling. This could be product images, user-generated content, or any visual data relevant to your business. Ensure that you have a sufficient quantity of data to train your models effectively.
Step 3: Train Your Team
Provide training for your team on how to use the selected tools effectively. This may include tutorials, workshops, or dedicated training sessions to enhance their labeling skills and ensure consistency.
Step 4: Establish Annotation Guidelines
Create detailed guidelines to maintain consistency in labeling across different team members. This should include specific definitions for categories, annotation methods, and quality control measures.
Step 5: Monitor and Evaluate
Set up a monitoring system to evaluate the effectiveness of your labeling efforts. Regularly review labeled data for accuracy and provide feedback to your team to continuously improve quality.
Common Challenges in Image Labeling
While image labeling is crucial for classification tasks, it also comes with its challenges. Here are some common issues businesses may face:
1. Time-Consuming Process
Labeling large volumes of images can be labor-intensive and time-consuming. Automation can help alleviate this burden, but it’s important to ensure that automated processes are accurate.
2. Ensuring Consistency
Diverse teams may have different interpretations of labeling guidelines, leading to inconsistencies. Regular training and clear guidelines can mitigate this issue.
3. Dealing with Ambiguity
Some images may be ambiguous or unclear, making labeling difficult. Providing clear examples and definitions for complex categories can help reduce confusion.
Conclusion
In conclusion, investing in a quality image labeling tool for classification is essential for businesses looking to leverage visual data efficiently. With the right tools and strategies in place, companies can enhance their data management processes, improve machine learning outcomes, and ultimately provide better services to their customers. The journey towards effective image classification begins with choosing the right labeling tool, establishing comprehensive guidelines, and continuously monitoring the quality of labeled data. Embrace the power of image labeling to propel your business forward in an increasingly competitive landscape.